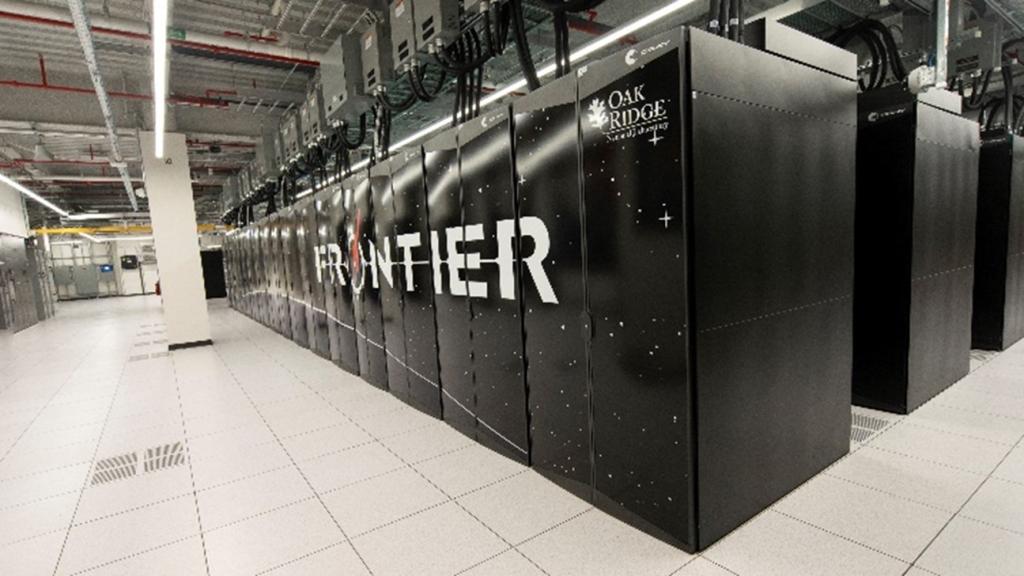
Research teams at the Department of Energy’s Oak Ridge National Laboratory received computing resource awards to train and test AI foundation models for science.
A total of six ORNL projects were awarded allocations from the National Artificial Intelligence Research Resource, or NAIRR, pilot and the Innovative and Novel Computational Impact on Theory and Experiment, or INCITE, program to train their AI models.
The NAIRR pilot is a concept for national infrastructure that bridges U.S.-based researchers with computational, data, software, model and training resources to further explore the applicability of AI in and outside a research environment. INCITE is a joint program between ORNL and Argonne National Laboratory designed to provide the scientific community with access to the nation’s fastest supercomputers for conducting high-impact research and simulations.
“ORNL’s AI Initiative projects in scientific foundation models represent a strong convergence of AI, supercomputing power and science,” said Prasanna Balaprakash, director of AI programs at ORNL. “By securing both NAIRR and INCITE computing awards, our teams are uniquely positioned to tackle the most complex scientific challenges in training, fine tuning and deploying foundation models for science.
“What distinguishes our approach is the integration of domain expertise with advanced scalable AI methodologies, creating foundation models to accelerate scientific discovery across disciplines,” Balaprakash added.
Xiao Wang, a research staff scientist in the Computational Science and Engineering Division at ORNL, along with ORNL Senior Computational Earth Scientist Dan Lu, and their team, was awarded two NAIRR allocations. They will use this opportunity to fine-tune and test their foundational models for energy transition and to operate supercomputers more efficiently.
“We are thrilled by this new opportunity and in this new allocation,” said Wang. “Working with these AI models require lots of data and lots of computing resources. The resources provided by the NAIRR pilot and NVIDIA will equip us with what we need to further our research and bring it to completion.”
The team plans to use one of its allocations to develop a vision transformer specifically designed for analyzing spatial-temporal data, which refers to information that includes both location and time, showing how something changes over space and time. “Our goal is to see how we can design the vision transformer architecture and also the training algorithm in a way that's computationally efficient and accurate when we train on a large supercomputer,” Wang said. One of the applications on which the model will be tested is cancer screening.
“We're going to test it on pathology imaging and segmentation to see if we can use this training method to develop a foundation model for pathology image and cancer segmentation for accurately identifying cancer when we train AI to look at different pathology images,” Wang added.
What distinguishes our approach is the integration of domain expertise with advanced scalable AI methodologies, creating foundation models to accelerate scientific discovery across disciplines.
The second allocation will be for the Oak Ridge Base Foundation Model for Earth System Predictability, or ORBIT, which was a Gordon Bell award finalist and won the 2024 Supercomputing Achievement award from HPC Wire.
“Our next step is to use this new allocation to further train and refine the accuracy of the model for Earth system predictability,” he said.
Dalton Lunga, who serves as group leader for GeoAI and a senior R&D staff scientist at ORNL, said the NVIDIA NAIRR award is intended to train spatially aware multimodal foundation models for Earth observation applications.
“The allocation will enable the project to explore a vast number of scientific experiments exploring varying spatial resolutions, non-concurrent sensor acquisitions, and pairing remote sensing (pixels and point-clouds), in-situ measurements (points), and georeferenced information to bind and enrich multimodal representations,” Lunga said. “The pretrained and finetuned models will support our scientific understanding of the built, physical and natural environment as well as rapid response monitoring of natural disasters.”
ORBIT will have an INCITE allocation as well, said Tsaris Aristeidis, research scientist in ORNL’s National Center for Computational Sciences.
"Last year, we developed the ORBIT foundation model for the Earth system predictability,” he said. “This year, supported by an INCITE allocation, we will advance ORBIT to deliver higher spatial resolution forecasts. These high-resolution, accurate forecasts will support critical multi-sector applications, including improving the reliability of energy generation and enhancing natural hazard mitigation efforts."
Pei Zhang, a computational scientist at ORNL, worked on a project to create a trustworthy foundation model to better understand turbulence. Called the Trustworthy Multi Scale Adaptive Turbulence Foundation Model, or MATEY, Zhang said this NAIRR allocation will allow them to have the computing hours needed to train such a large-scale model.
“With all these computing hours together, they’ll help us to build this large foundation model,” Zhang said. “Then, we can send this model to other scientists, and they can use our model in their applications.”
Another NAIRR recipient is a project led by Amir Ziabari, senior R&D staff data scientist, focused on advancing 3D imaging using artificial intelligence. The team is developing a new type of AI model called DiffusiveINR, which combines two cutting-edge techniques: one that generates images by gradually removing noise, and another that represents 3D objects more smoothly and efficiently than traditional methods. This model will help scientists reconstruct high-resolution 3D images from limited or incomplete data — important for applications in materials science, healthcare, and manufacturing.
Integrating these advanced algorithms into the DiffusiveINR foundational model allows for 3D reconstruction from sparse and incomplete scientific imaging data commonly being captured at various centers and facilities at ORNL.
Funding for these projects was made possible through ORNL’s AI Initiative and Laboratory Directed Research and Development. ORNL’s Oak Ridge Leadership Computing Facility is a DOE Office of Science user facility and home to the Frontier supercomputer, the world’s fastest for open science and the first to break the exascale barrier.
UT-Battelle manages ORNL for the Department of Energy’s Office of Science, the single largest supporter of basic research in the physical sciences in the United States. The Office of Science is working to address some of the most pressing challenges of our time. For more information, please visit energy.gov/science. — Mark Alewine